
Adoption of Multi-Cloud and Hybrid Cloud Strategies: The Smart Move for Modern Enterprises
In today’s digital-first world, businesses are no longer relying on a single cloud provider to meet their evolving needs. Instead, they are adopting multi-cloud and hybrid cloud strategies to optimize performance, enhance flexibility, and ensure business continuity. But navigating this complex cloud ecosystem requires smart orchestration, automation, and governance—and that’s where PurpleCube AI comes in.
Adoption of Multi-Cloud and Hybrid Cloud Strategies: The Smart Move for Modern Enterprises
In today’s digital-first world, businesses are no longer relying on a single cloud provider to meet their evolving needs. Instead, they are adopting multi-cloud and hybrid cloud strategies to optimize performance, enhance flexibility, and ensure business continuity. But navigating this complex cloud ecosystem requires smart orchestration, automation, and governance—and that’s where PurpleCube AI comes in.
Multi-Cloud vs. Hybrid Cloud: What’s the Difference?
Before diving into why businesses are adopting these strategies, let’s break them down:
🔹 Multi-Cloud – Using two or more cloud providers (AWS, Azure, Google Cloud) to distribute workloads and avoid vendor lock-in.
🔹 Hybrid Cloud – A mix of public and private cloud environments working together to balance scalability and security.
Both approaches empower enterprises with more control, resilience, and performance optimization, but they also introduce new challenges in data management, integration, and security.
Why Are Businesses Moving to Multi-Cloud & Hybrid Cloud?
1️ Avoiding Vendor Lock-In
Relying on a single cloud provider can be risky. Multi-cloud adoption ensures businesses can switch providers if pricing, service quality, or compliance needs change.
2️ Enhancing Resilience & Business Continuity
A hybrid and multi-cloud setup mitigates risks by distributing workloads across platforms, ensuring that outages in one cloud provider don’t cripple business operations.
3 Optimizing Costs & Performance
Different workloads require different environments. Companies can leverage cost-effective cloud solutions based on performance and storage needs, optimizing their cloud spending.
4️ Regulatory & Compliance Flexibility
Certain industries have strict data sovereignty laws. A hybrid approach allows businesses to keep sensitive data in a private cloud while using public clouds for scalability and computing power.
5️ AI & Data Workload Efficiency
With AI and analytics workloads growing, businesses need flexible infrastructure to handle real-time processing, data orchestration, and model training across multiple clouds.
Challenges in Multi-Cloud & Hybrid Cloud Adoption
While the benefits are significant, managing multiple cloud environments presents challenges:
🔸 Data Silos & Integration Complexities – Ensuring seamless data flow across cloud environments.
🔸 Security & Compliance Risks – Consistently enforcing security policies across different providers.
🔸 Cost & Performance Management – Optimizing cloud costs while maintaining high performance.
🔸 Governance & Automation – Maintaining data quality, lineage, and governance across diverse platforms.
How do businesses overcome these challenges? By leveraging intelligent cloud-native solutions that automate and simplify cloud management.
How PurpleCube AI Powers Multi-Cloud & Hybrid Cloud Success
At PurpleCube AI, we enable businesses to seamlessly orchestrate, automate, and optimize their multi-cloud and hybrid cloud strategies.
✅ AI-Driven Data Orchestration – Unify and automate data pipelines across AWS, Azure, and Google Cloud.
✅ Built-in Governance & Compliance – Ensure data security, lineage, and compliance across multiple environments.
✅ Cost Optimization & Performance Monitoring – Smart insights to help businesses reduce cloud costs while maintaining performance.
✅ Low-Code Cloud Integration – Connect, transform, and deploy data effortlessly—without deep technical expertise.
💡 The future is multi-cloud and hybrid. The key to success is choosing a platform that simplifies cloud adoption, enhances automation, and ensures compliance—and that’s what PurpleCube AI delivers.
🚀 Ready to Elevate Your Cloud Strategy?
Explore how PurpleCube AI can help you unlock the full potential of multi-cloud and hybrid cloud strategies—without the complexity.
👉 Let’s Talk! Contact us today. Try for Free

Machine Learning and Artificial Intelligence Integration: Powering the Next Wave of Innovation
In today’s digital era, the terms Artificial Intelligence (AI) and Machine Learning (ML) are often used interchangeably, but they're not the same. While AI refers to the broader concept of machines performing tasks in a way that we consider “smart,” ML is a specific subset that enables systems to learn and improve from data without explicit programming.
Machine Learning and Artificial Intelligence Integration: Powering the Next Wave of Innovation
In today’s digital era, the terms Artificial Intelligence (AI) and Machine Learning (ML) are often used interchangeably, but they're not the same. While AI refers to the broader concept of machines performing tasks in a way that we consider “smart,” ML is a specific subset that enables systems to learn and improve from data without explicit programming.
When seamlessly integrated, AI and ML create a powerful synergy, enabling organizations to build systems that don’t just automate tasks, but continuously learn, adapt, and evolve. This integration is transforming industries, driving innovation, and unlocking unprecedented opportunities.
AI and ML: Better Together
Think of AI as the brain and ML as the learning mechanism. AI defines the goals, decision-making, reasoning, problem-solving, while ML supplies the brain with data-driven intelligence, helping it get smarter over time.
Together, they power a range of capabilities:
- Predictive analytics in healthcare and finance
- Natural language processing in virtual assistants
- Computer vision in manufacturing and automotive
- Anomaly detection in cybersecurity and telecom
By integrating ML into AI systems, businesses create solutions that don’t just follow rules, they identify patterns, anticipate outcomes, and recommend actions based on real-time insights.
Real-World Impact: From Automation to Augmentation
This integration has already started reshaping the enterprise landscape:
🔹 Customer Experience: Personalized recommendations on streaming platforms and e-commerce sites rely on ML-driven AI to understand user behavior and optimize content delivery.
🔹 Healthcare: AI agents assist doctors by analyzing vast medical data sets, while ML models continuously improve diagnostic accuracy.
🔹 Supply Chain: Integrated systems predict demand, optimize routes, and respond to disruptions with agility.
The goal isn’t to replace humans, it’s to augment their capabilities, freeing them from routine decisions and enabling them to focus on strategic and creative tasks.
Challenges and Considerations
As powerful as AI-ML integration is, it comes with challenges:
- Data quality and availability: Garbage in, garbage out. ML needs clean, diverse, and relevant data.
- Model interpretability: Black-box algorithms can be risky, especially in regulated industries.
- Ethical concerns: Bias in data can lead to biased outcomes. Responsible AI practices are essential.
- Scalability: Integrating ML into AI systems at scale requires robust infrastructure and continuous monitoring.
Successful integration requires not just technology, but the right governance, skill sets, and mindset.
The Road Ahead: Smarter Systems, Better Outcomes
As we look to the future, the integration of ML and AI will drive the evolution of autonomous systems, from self-driving vehicles to intelligent enterprise agents. With advances in deep learning, reinforcement learning, and neural networks, AI will become more contextual, proactive, and human-like in its decision-making.
Organizations that embrace this shift today will be better equipped to innovate, compete, and lead tomorrow.
AI + ML = The Future, Now Powered by PurpleCube AI
Machine Learning and Artificial Intelligence, when integrated, are not just enhancing processes, they’re reinventing the way businesses think, act, and grow. From smarter decisions to proactive operations, the AI+ML duo is shaping a future where adaptability, speed, and intelligence define success.
At PurpleCube AI, we make this future accessible.
With our GenAI-enabled data orchestration platform, businesses can seamlessly harness the power of AI and ML to build intelligent, real-time, and scalable data workflows. Whether you're streamlining operations, enhancing customer experiences, or predicting future outcomes, PurpleCube AI empowers you to do it all, effortlessly.
Ready to turn your data into action? Discover what's possible with PurpleCube AI. Try for Free

The Rise of DataOps for Enhanced Analytical Efficiency
In today's rapidly changing data landscape, organizations are moving beyond simply gathering information to focusing on deriving actionable insights. Analytical efficiency has emerged as a fundamental element of contemporary decision-making, necessitating more than just advanced tools and technologies. This is where DataOps comes into play—a groundbreaking methodology aimed at optimizing the entire data lifecycle, from data ingestion to actionable insights. But what is DataOps, and how is it reshaping analytical efficiency? Let's explore further.
The Rise of DataOps for Enhanced Analytical Efficiency
In today's rapidly changing data landscape, organizations are moving beyond simply gathering information to focusing on deriving actionable insights. Analytical efficiency has emerged as a fundamental element of contemporary decision-making, necessitating more than just advanced tools and technologies. This is where DataOps comes into play—a groundbreaking methodology aimed at optimizing the entire data lifecycle, from data ingestion to actionable insights. But what is DataOps, and how is it reshaping analytical efficiency? Let's explore further.
At its essence, DataOps promotes communication and collaboration among data engineers, analysts, scientists, and business stakeholders. By dismantling silos and streamlining workflows, it empowers organizations to fully leverage their data.
Key Pillars of DataOps
- Collaboration: DataOps thrives on teamwork across various functions. It aligns data teams with business objectives, ensuring that all efforts are directed toward achieving meaningful results.
- Automation: Routine tasks, such as data validation and pipeline deployment, are automated to minimize errors and boost efficiency.
- Continuous Delivery: DataOps focuses on delivering small, incremental updates to data pipelines, keeping analytics current.
- Monitoring and Feedback: Real-time monitoring of data workflows and ongoing feedback loops help pinpoint bottlenecks and enhance processes.
- Data Quality: Maintaining clean, consistent, and reliable data is a fundamental principle of DataOps.
The Role of DataOps in Analytical Efficiency
- Accelerating Insights: Traditional data workflows often involve prolonged cycles of data preparation, validation, and analysis. DataOps streamlines these cycles through automation and collaboration, facilitating quicker insights. Businesses can swiftly adapt to market changes and customer demands, gaining a competitive advantage.
- Enhancing Data Quality: Poor data quality can undermine analytics. DataOps incorporates rigorous testing frameworks and validation checks at every stage of the pipeline, ensuring that analysts and decision-makers can rely on the data.
- Streamlining Data Pipelines: With DataOps, pipelines become flexible and modular, allowing for adjustments on the fly. This adaptability ensures seamless operations as data sources, volumes, and requirements evolve.
- Reducing Time-to-Value: By applying continuous integration/continuous deployment (CI/CD) principles, DataOps significantly shortens the time needed to convert raw data into actionable insights. Teams can concentrate on high-value tasks rather than addressing operational inefficiencies.
- Bridging the Gap Between IT and Business: One of the key advantages of DataOps is its ability to align technical teams with business goals. This alignment guarantees that data initiatives are not only technically sound but also strategically significant.
Key Trends Driving DataOps Adoption
- Explosion of Data Sources: As organizations embrace IoT devices, cloud platforms, and third-party integrations, managing diverse data sources becomes increasingly complex. DataOps offers a structured framework to navigate this complexity effectively.
- Rise of Real-Time Analytics: The demand for real-time insights is at an all-time high. DataOps enables the creation of pipelines that process and deliver data instantaneously, catering to applications like fraud detection and predictive maintenance.
- AI and Machine Learning Integration: DataOps accelerates AI/ML workflows by ensuring that models are trained on high-quality, up-to-date data. This integration enhances model accuracy and expedites deployment.
- Focus on Data Governance: With stricter regulations such as GDPR and CCPA, organizations must prioritize data governance. DataOps integrates governance practices into workflows, ensuring compliance without sacrificing agility.
Challenges in Implementing DataOps
- Cultural Resistance: Transitioning from siloed operations to a collaborative DataOps model necessitates a cultural shift that some organizations may find challenging.
- Skill Gaps: Successfully implementing DataOps requires a blend of technical and analytical skills. Upskilling teams and recruiting DataOps specialists can be resource-intensive.
- Tool Integration: Merging various tools and platforms into a cohesive DataOps workflow can be complex, particularly for organizations with legacy systems.
The Future of DataOps
As businesses increasingly prioritize data-driven strategies, DataOps is set to become a standard practice. Emerging technologies such as automated data observability, AI-driven pipeline optimization, and serverless data architectures will further enhance its capabilities. Organizations that adopt DataOps will not only achieve analytical efficiency but also position themselves as leaders in their respective industries.
Conclusion
The rise of DataOps signifies a transformative shift in how organizations manage and analyze data. By promoting collaboration, automating workflows, and emphasizing data quality, DataOps ensures that businesses can extract maximum value from their data assets. For organizations striving to remain competitive in a data-driven landscape, embracing DataOps is not merely an option—it is essential.
Are you prepared to revolutionize your analytics with DataOps? Discover how Edgematics advanced solutions, PurpleCube AI, can assist you in implementing DataOps and achieving unmatched analytical efficiency. Contact us today to learn more!
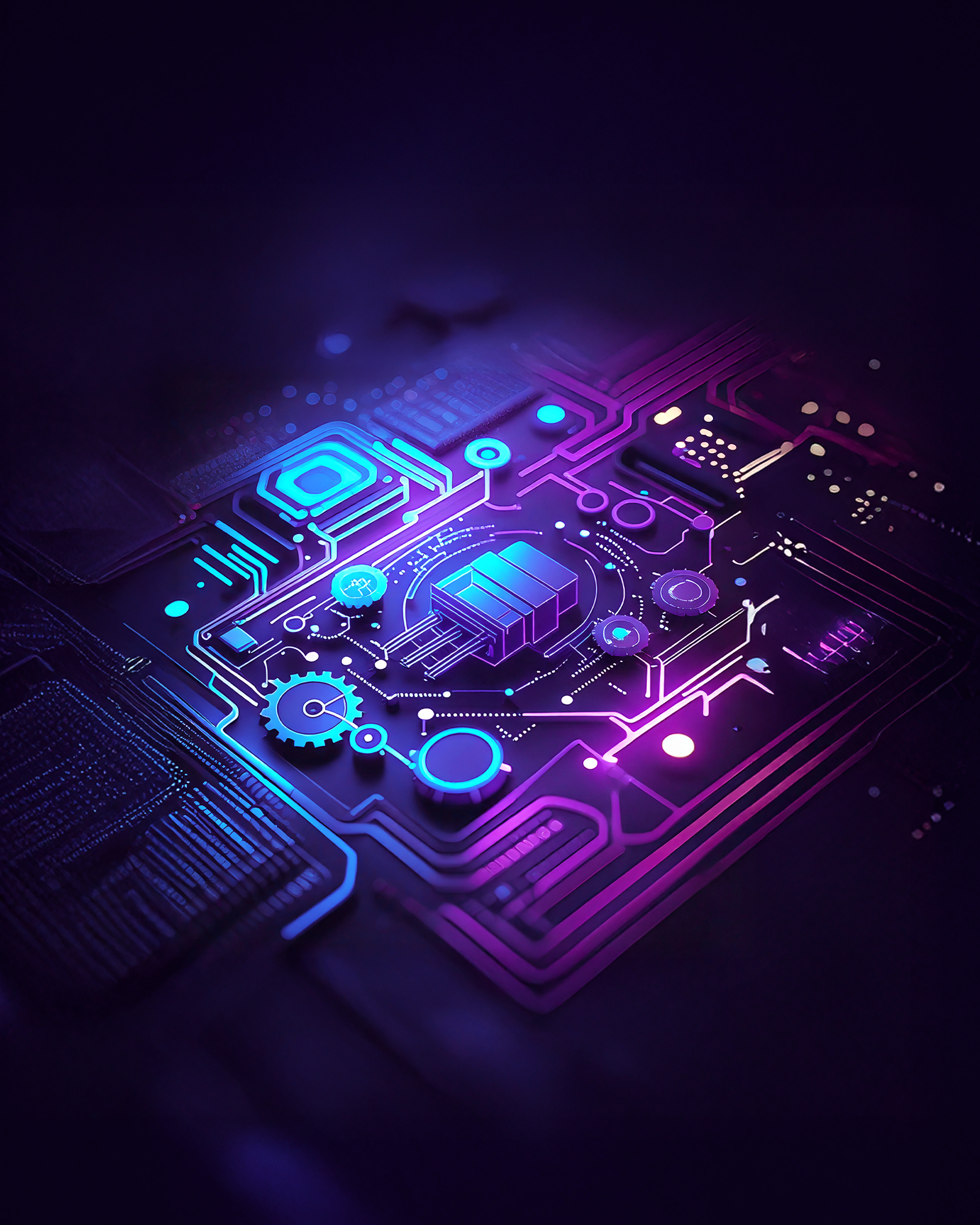
The Backbone of Automation: How Connectors Simplify Enterprise Workflows
In the rapidly evolving landscape of modern enterprises, automation stands as a cornerstone of efficiency, agility, and innovation. At the core of every effective automated process are connectors—powerful tools that bridge the divide between disparate systems, applications, and data sources. By leveraging connectors, organizations can unlock the full potential of their workflows. This document explores the role of connectors in simplifying enterprise workflows and highlights their essential nature in the current automation ecosystem.
In the rapidly evolving landscape of modern enterprises, automation stands as a cornerstone of efficiency, agility, and innovation. At the core of every effective automated process are connectors—powerful tools that bridge the divide between disparate systems, applications, and data sources. By leveraging connectors, organizations can unlock the full potential of their workflows. This document explores the role of connectors in simplifying enterprise workflows and highlights their essential nature in the current automation ecosystem.
The Need for Connectors in Enterprise Workflows
Modern enterprises utilize a wide range of tools to manage their operations, from project management and customer relationship management (CRM) to finance and supply chain systems. However, the multitude of applications can create data silos, inefficiencies, and bottlenecks. Connectors effectively address these challenges by:
- Enabling Data Flow: Ensuring seamless data movement between systems, reducing duplication and inconsistencies.
- Improving Collaboration: Fostering cross-departmental collaboration by uniting tools.
- Enhancing Decision-Making: Providing real-time data integration for quicker, informed decisions.
Key Benefits of Connectors in Automation
- Streamlining Operations:
Manual data entry and siloed systems can slow down processes and increase error risks. Connectors automate repetitive tasks, making workflows more streamlined and reliable.
- Boosting Efficiency:
By automating data exchange between applications, connectors eliminate redundancies, allowing employees to focus on strategic tasks. For example, syncing sales data from CRM to accounting systems reduces manual intervention.
- Enhancing Scalability:
As businesses expand, their workflows become more complex. Connectors enable enterprises to scale without overhauling existing systems, ensuring compatibility and integration across platforms.
- Reducing Costs:
Automation through connectors minimizes the need for custom integrations, lowering development costs and maintenance overhead.
- Improving User Experience:
Connectors allow users to access data and functionalities from multiple systems within a single interface, enhancing productivity and satisfaction.
Real-World Applications of Connectors
- E-Commerce: Connect inventory management systems with online stores to automate stock updates and order processing.
- Marketing: Sync marketing automation platforms with CRMs to streamline lead nurturing and campaign tracking.
- Finance: Integrate accounting software with payroll systems to automate financial reporting and compliance.
- Healthcare: Connect electronic medical records (EMRs) with analytics tools to provide actionable insights for patient care.
Challenges Connectors Address
- Eliminating Data Silos:
Data silos hinder efficiency and innovation. Connectors break down these barriers, fostering a unified data ecosystem.
- Ensuring Real-Time Updates:
In dynamic environments, outdated information can lead to poor decisions. Connectors enable real-time synchronization, ensuring accurate and up-to-date data.
- Simplifying Integration:
Building custom integrations for every system is time-consuming and costly. Connectors provide plug-and-play solutions that simplify the integration process.
Why Connectors Are the Backbone of Automation
The success of automation relies on creating cohesive workflows across a diverse tech stack. Connectors serve as the backbone of this integration, transforming fragmented systems into a unified, agile ecosystem. By enabling interoperability, they empower organizations to:
- Accelerate digital transformation.
- Enhance operational agility.
- Drive innovation through data-driven decisions.
Embracing the Future with Edgematics
PurpleCube AI specializes in facilitating seamless enterprise automation through its advanced low-code data orchestration platform. With an extensive library of connectors, PurpleCube AI bridges the gap between your systems, eliminating silos and enhancing efficiency. Whether your goal is to streamline operations, improve collaboration, or accelerate decision-making, PurpleCube AI ensures your workflows are as efficient and scalable as your ambitions.
Unlock the power of connectors and elevate your automation efforts with PurpleCube AI. Contact us today to discover how we can transform your enterprise workflows.

Eckerson Group - Data Orchestration for the Modern Enterprise
PurpleCube AI offers a data orchestration platform that unifies and streamlines data engineering across data centers, regions, and clouds. It helps enterprises reduce their number of pipeline tools, accelerate performance, and reduce cost as they support business intelligence (BI) and artificial intelligence and machine learning (AI/ML) projects as well as operational workloads. PurpleCube AI targets three primary use cases: data modernization, data preparation, and self-service. It differentiates its platform with cost performance, generative AI assistance, a unified platform, flexibility, and active metadata. Eckerson Group recommends that data and analytics leaders evaluate how PurpleCube AI might alleviate their data silos and pipeline bottlenecks; learn more about PurpleCube AI’s differentiators; and compare PurpleCube AI to alternative approaches with both current requirements and likely future requirements in mind.
PurpleCube AI
Data Orchestration for the Modern Enterprise
This publication may not be reproduced or distributed without Eckerson Group’s prior permission.
About the Author

Kevin Petrie is VP of Research at Eckerson Group, where he manages the research agenda and writes independent reports about topics such as data integration, generative AI, machine learning, and cloud data platforms. For 25 years Kevin has deciphered what technology means for practitioners as an industry analyst, instructor, marketer, services leader, and journalist. He launched a global data analytics services team for EMC Pivotal and ran field training at the data integration provider Attunity (now part of Qlik). A frequent public speaker and coauthor of two books about data management, Kevin loves helping start-ups educate their communities about emerging technologies.
About Eckerson Group
Eckerson Group is a global research, consulting, and advisory firm that helps organizations get more value from data. Our experts think critically, write clearly, and present persuasively about data analytics. They specialize in data strategy, data architecture, self-service analytics, master data management, data governance, and data science. Organizations rely on us to demystify data and analytics and develop business-driven strategies that harness the power of data. Learn what Eckerson Group can do for you!
About This Report
Eckerson Group provides independent and objective research on emerging technologies, techniques, and trends in the field. Although we do not recommend vendors or products, we write sponsored profiles such as this one to help practitioners understand different offerings.
Executive Summary
PurpleCube AI offers a data orchestration platform that unifies and streamlines data engineering across data centers, regions, and clouds. It helps enterprises reduce their number of pipeline tools, accelerate performance, and reduce cost as they support business intelligence (BI) and artificial intelligence and machine learning (AI/ML) projects as well as operational workloads. PurpleCube AI targets three primary use cases: data modernization, data preparation, and self-service. It differentiates its platform with cost performance, generative AI assistance, a unified platform, flexibility, and active metadata. Eckerson Group recommends that data and analytics leaders evaluate how PurpleCube AI might alleviate their data silos and pipeline bottlenecks; learn more about PurpleCube AI’s differentiators; and compare PurpleCube AI to alternative approaches with both current requirements and likely future requirements in mind.
Company
CEO Bharat Phadke cofounded PurpleCube AI in 2020 to help enterprises tackle the persistent problem of siloed, bottlenecked data. As leader of the consulting firm Edgematics, Phadke had witnessed this problem firsthand. His clients encountered project delays and cost overruns as they tried to manage heterogeneous data lakes with multiple data pipeline tools. To alleviate that pain, Phadke and his team built a data orchestration platform that unifies and streamlines data engineering across data centers, regions, and clouds. PurpleCube AI’s platform helps enterprises improve the cost performance of extract, load, and transform (ELT) workloads, with the flexibility to choose where to process their data. Today this bootstrapped venture helps Fortune 2000 companies simplify how they manage data in hybrid, cloud, and multi-cloud environments. PurpleCube AI offers a graphical user interface (GUI), generative AI (GenAI) chatbot, and distributed architecture to help data engineers and architects—or even business analysts—prepare data for analytics. It extracts data from a myriad of sources, then loads and transforms it on targets that include Cloudera, the Databricks lakehouse, Snowflake Data Cloud, and hyperscalers such as AWS or Microsoft Azure. PurpleCube AI users can validate data quality, perform exploratory analysis, and orchestrate pipelines with scheduling and monitoring capabilities. Enterprises use PurpleCube AI to boost productivity and efficiency as they democratize data consumption throughout the organization.
Figure 1. PurpleCube AI Data Orchestration Platform

Figure 1 illustrates the range of PurpleCube AI capabilities for modern data ecosystems.
Target Customers and Use Cases
PurpleCube AI’s ideal customer is an enterprise that struggles to deliver multi-sourced data to business intelligence (BI) projects, artificial intelligence/machine learning (AI/ML) projects, and operational applications. Its data teams use different pipeline tools for different parts of their environment, reducing productivity and exacerbating data silos. These data engineers and architects fall short of cost performance objectives with heritage Hadoop data lakes in particular. Short-staffed, they cannot keep pace with business demands for structured and semistructured data from various sources. They need to consolidate tools, reduce scripting, and accelerate pipelines while also enabling self-service for a growing population of business-oriented data consumers.
Customers such as Virgin Mobile and Canadian Tyre implement PurpleCube AI to meet such objectives. Data managers and consumers alike use PurpleCube AI to manipulate data sets with spoken language, mouse clicks, or command lines, according to their preference. They build, orchestrate, and optimize high-performance data pipelines that span many sources, targets, and processing engines. These pipelines can transfer data in batches or streams across software-as-a-service (SaaS) applications, file systems, databases, data warehouses, and lakehouses. PurpleCube AI unifies such disparate elements with automated workflows to help companies better support analytics and operations.
PurpleCube AI targets three primary use cases: data modernization, data preparation, and self-service.
Data modernization. Enterprises use PurpleCube AI to modernize their data architectures. With its help, data engineers and architects can perform initial migrations as well as subsequent updates, using continuous integration and continuous delivery (CI/CD) capabilities to refine pipelines, change sources, and tune performance. Capabilities such as these enable enterprises to transform and validate data in support of cloud-based analytical projects and operational applications. T-Mobile, for example, uses PurpleCube AI to move data to Snowflake.
Data preparation. Data engineers use PurpleCube AI to simplify data preparation. The PurpleCube AI studio enables them to configure, schedule, and monitor the pipelines that transform data for analytics. They can discover tables or other data objects, then reformat, merge, cleanse, filter, and structure them to meet consumption requirements. With the help of GenAI, data teams can query and explore these data sets, generate quality rules, and enrich metadata. Data engineers at Home Depot use PurpleCube AI to consolidate purchase records on Google BigQuery, then synchronize dynamic source schemas to prepare for real-time and historical customer analytics.
Self-service. Data analysts, data scientists, and business analysts use PurpleCube AI to prepare data for analytics themselves rather than relying on busy data engineers. They design pipelines in the studio, then inspect, analyze, and share the resulting data product. PurpleCube’s GenAI capabilities play a major role in self-service, helping less technical users prepare data and query tables on their own. They can explore, inspect, and interpret data using their business knowledge, with no little or no need for scripting skills.
Product Functionality
All these data and business stakeholders collaborate on the PurpleCube AI platform to integrate data for analytics and operations, using the PurpleCube AI Studio as well as additional capabilities.
PurpleCube AI Studio creates and assembles the components of a pipeline. Its primary elements are data points, data objects, data flows, job flows, and data connect (see Figure 2).
Figure 2. PurpleCube AI Studio

> Data points provide connection details for source and target systems, such as hostname, IP address, and user credentials. They also help standardize data transfer.
> Data objects encapsulate metadata—names, schemas, attributes, and so on—that describes physical objects such as tables, views, and files.
> Data flows are the ELT pipelines that use data points and data objects to map attributes between sources and targets.
> Job flows define the sequences of jobs that a pipeline executes, including their order, dependencies, and triggers.
> Data connect performs real-time ingestion via change data capture technology and integration with message brokers such as Apache Kafka.
Figure 3 illustrates a sample data pipeline based on these elements.
Figure 3. Configuring a Data Pipeline
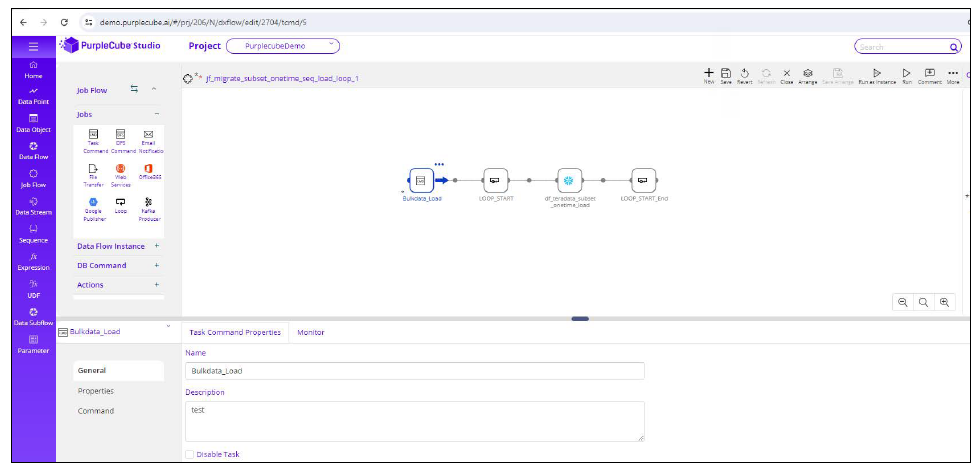
Additional capabilities
Additional product capabilities include the monitor, scheduler, SQL editor, GenAI module, admin, data stream, and command line interface (CLI).
> The monitor tracks job status and operational metrics, with visual displays and alerts as well as debugging assistance.
> The scheduler enables the user to specify task execution dates, times, and frequency, as well as events that trigger tasks.
> The SQL editor enables users to discover and explore data, eliminating the need for a separate SQL client. It provides views of tables and runs custom queries.
> The GenAI module provides a chatbot through which users instruct PurpleCube AI to perform commands based on their choice of language model (GPT or Vertex to start).
> The admin enables administrators to set up and control project parameters, application settings, user privileges, agent configurations, and connectivity settings.
> The data stream uses Spark to perform real-time data transformations on file systems or object stores.
> The command line interface (CLI) receives and executes instructions from Linux machines or applications that use REST APIs.
Differentiators
PurpleCube AI competes with data pipeline vendors such as Informatica and Talend (now part of Qlik). It differentiates its offering with cost performance, GenAI assistance, a unified platform, flexibility, and active metadata.
Cost performance. PurpleCube AI optimizes workloads to improve the cost performance of data management in several ways. Its distributed agents push the workload down to local processing engines such as Apache Spark for Databricks, massively parallel processors for Teradata, and so on. It also places the data in optimally sized files using the Hadoop file system (HDFS) during the processing phase to make the most efficient use of CPU and memory. This lightweight footprint and approach helps increase throughput, decrease latency, and reduce cost. PurpleCube AI helped Scotia Bank, for example, reduce tool and infrastructure costs by $1.5 million while consolidating data to detect money laundering.
GenAI assistance. The GenAI module, in beta as of Q1 2024, both increases productivity for technical users and enables self-service for business users. On the technical side, data engineers and data analysts can type natural language commands to autogenerate queries, data quality rules, or descriptions of glossary terms. Such capabilities help these technical users complete projects faster and free up time for additional projects. Business analysts, meanwhile, can use the GenAI module to discover, format, and query data as part of exploratory analysis. This helps democratize data consumption by making insights available to more business users without increasing the workload for data engineers.
Unified platform. PurpleCube AI unifies the ingestion, transformation, and validation of structured and semistructured data sets to support various types of workloads. It integrates with more than 150 sources and targets to ingest data in periodic batches or real-time increments. These broad capabilities enable PurpleCube AI users to consolidate pipeline tools and gain productivity, simplifying data management even as the underlying data sources and targets proliferate. Later this year, PurpleCube AI plans to add support for semi- or unstructured data objects such as the text and images that feed new generative AI initiatives.
Flexibility. PurpleCube AI offers flexibility on several dimensions. For starters, customers can process data on their choice of existing infrastructure rather than having to configure, implement, or purchase separate compute resources. PurpleCube AI also supports a wide range of workloads, platforms, and users thanks to open APIs and data formats. Users can consume and interact with data themselves, for example using GenAI, rather than pulling up a standalone BI tool. In addition, they can port PurpleCube AI software between processing engines without rewriting code or reconfiguring systems. Finally, customers say PurpleCube AI’s services team speeds implementations, reduces training requirements, and rapidly resolves issues.
Active metadata. PurpleCube AI uses metadata to optimize processes and simplify the user experience. It gathers the metadata that describes users, tasks, events, systems, pipeline code, and applications. This rich metadata enables PurpleCube AI’s controller to give efficient instructions to agents and orchestrate workflows across the elements of enterprise environments. Metadata also streamlines workloads by enabling PurpleCube AI to optimize file sizes and consumption of CPU or memory resources. Users have open access to all this metadata, which gives them graphical views of data lineage to assist impact analysis and governance programs. By going further to expose its code and metadata, PurpleCube AI helps developers build their own extensions in less time.
Architecture
A browser-based user interface, which includes the studio, monitor, admin, and scheduler, issues instructions to a Java-based controller. This controller serves as the brain of PurpleCube AI. It captures user instructions, maintains them in the metadata repository, and compiles them into messages that Java-based agents then execute on pipelines, sources, and targets. A broker manages communications between agents and the controller (see Figure 4).
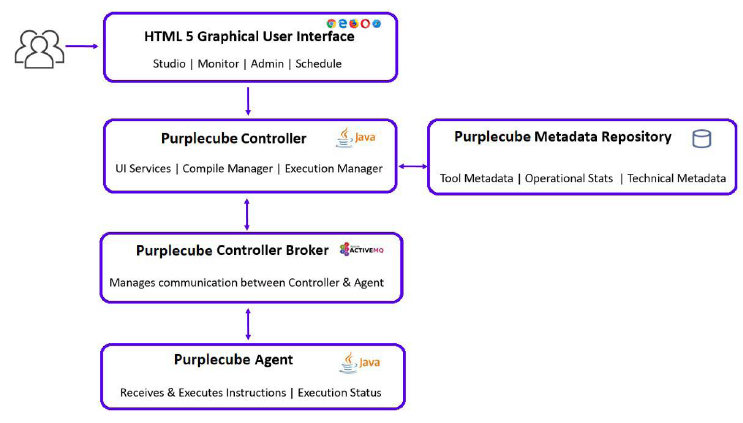
As described earlier, PurpleCube AI pushes data processing workloads down to underlying platforms such as Hadoop, Teradata, Snowflake, Amazon Redshift, or Google BigQuery.
Pricing
PurpleCube AI offers express, advanced, and enterprise pricing plans:
> The express plan includes a single-user license for data ingestion across a limited number of sources and targets, along with five days of deployment consulting and 40 weekday hours of product support.
> The advanced plan includes three user licenses, support for more sources and targets, data cleansing, 10 days of deployment consulting, and 40 weekday hours of support.
> The enterprise plan comprises five user licenses, full source and target support, three custom connections, multi-environment support, and additional capabilities. It includes 15 days of deployment consulting and 24-7 support.
PurpleCube AI also offers a 30-day free trial with 24-7 support, and is available in all three cloud marketplaces.
Summary and Recommendations
PurpleCube AI’s data orchestration platform helps enterprises improve the cost performance of ELT workloads while choosing their own processing engine. PurpleCube AI offers a graphical user interface, GenAI chatbot, and distributed architecture to help data engineers and architects—or even business analysts—prepare data for analytics. It helps enterprises consolidate pipeline tools as they support BI, AI/ML, and operational workloads. PurpleCube AI targets three primary use cases: data modernization, data preparation, and self-service.
Eckerson Group recommends that data and analytics leaders take the following actions:
> Evaluate how PurpleCube AI might alleviate data silos and pipeline bottlenecks in their heterogeneous environments. They might find that PurpleCube AI can help them consolidate their tools and processes for data engineering.
> Learn more about PurpleCube AI’s differentiators—including cost performance, GenAI assistance, a unified platform, flexibility, and active metadata—and map those differentiators to their own data requirements.
> Compare PurpleCube AI to alternative approaches, including existing homegrown or commercial tools, in terms of its ability to meet both current requirements and likely future requirements.
About Eckerson Group
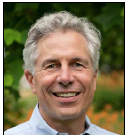
Wayne Eckerson, a globally known author, speaker, and consultant, formed Eckerson Group to help organizations get more value from data and analytics. His goal is to provide organizations with expert guidance during every step of their data and analytics journey.
Eckerson Group helps organizations in three ways:
> Our thought leaders publish practical, compelling content that keeps data analytics leaders abreast of the latest trends, techniques, and tools in the field.
> Our consultants listen carefully, think deeply, and craft tailored solutions that translate business requirements into compelling strategies and solutions.
> Our advisors provide competitive intelligence and market positioning guidance to software vendors to improve their go-to-market strategies.
Eckerson Group is a global research, consulting, and advisory firm that focuses solely on data and analytics. Our experts specialize in data governance, self-service analytics, data architecture, data science, data management, and business intelligence.
Our clients say we are hardworking, insightful, and humble. It all stems from our love of data and our desire to help organizations turn insights into action. We are a family of continuous learners, interpreting the world of data and analytics for you.
Get more value from your data. Put an expert on your side. Learn what Eckerson Group can do for you!
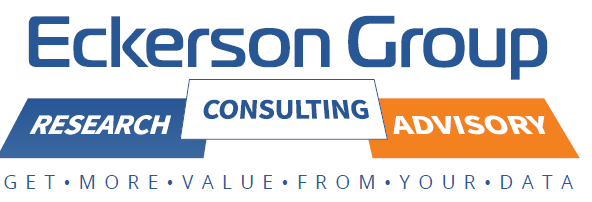
About the Sponsor
PurpleCube AI is a unified data orchestration platform that revolutionizes how businesses manage and utilize their data. We achieve this by directly embedding the power of Generative AI into the data engineering process. This unique approach enables us to:
Unify data engineering: PurpleCube AI provides a single platform to manage all your data engineering needs, from structured and semi-structured data to streaming data. This eliminates the complexity and cost associated with managing multiple tools and eliminates the need for specialized skills for each platform.
Automate complex tasks: Leveraging cutting-edge AI, PurpleCube AI allows you to streamline data integration, transformation, and processing.
Activate insights: PurpleCube AI empowers you to unlock valuable insights from your data with complete certainty and agility. Its comprehensive metadata management ensures data quality and trust, while its intelligent agents enable you to adapt and innovate at the speed of business.
Beyond standard data lake and warehouse automation, PurpleCube AI harnesses the power of language models. This enables a range of innovative use cases, including processing various file formats, uncovering hidden insights through exploratory data analysis and natural language queries, automatically generating and enriching metadata, assessing and improving data quality, and optimizing data governance through relationship modeling.
PurpleCube AI is ideal for:
> Data architects
> Data engineers
> Data scientists
> Data analysts
Across various industries:
> Banking
> Telecommunications
> Automotive
> Healthcare
> Retail
PurpleCube AI represents a true paradigm shift in data orchestration. We believe in empowering businesses to extract maximum value from their data, driving innovation, and achieving a competitive edge in the data-driven world.

Data Engineering and Data Governance: Elevating Your Data Team's Productivity, Efficiency, and Accuracy
In today’s data-centric business landscape, the combination of data engineering and data governance has become crucial for organizations striving to maximize the value of their data assets. By harmonizing robust data engineering practices with comprehensive governance frameworks, businesses can significantly enhance their data teams' productivity, efficiency, and accuracy. This synergy unlocks the true potential of data, empowering better decision-making and fostering a competitive edge.
Data Engineering and Data Governance: Elevating Your Data Team's Productivity, Efficiency, and Accuracy
In today’s data-centric business landscape, the combination of data engineering and data governance has become crucial for organizations striving to maximize the value of their data assets. By harmonizing robust data engineering practices with comprehensive governance frameworks, businesses can significantly enhance their data teams' productivity, efficiency, and accuracy. This synergy unlocks the true potential of data, empowering better decision-making and fostering a competitive edge.
Data engineers ensure that data flows seamlessly from its source to a central repository like a data warehouse or data lake, making it ready for analysis.
Key components include:
- Data Integration: Consolidating data from various sources into a unified system.
- Data Transformation: Cleaning and reshaping raw data for usability.
- Data Storage: Choosing appropriate storage solutions tailored to organizational needs, such as cloud databases or on-premise systems.
- Data Quality Assurance: Implementing measures to maintain accuracy, consistency, and reliability across datasets.
The Role of Data Governance
Data governance encompasses the management of data's availability, usability, integrity, and security within an organization. It sets the standards and processes that guide data handling, ensuring compliance and building trust in data assets. Core elements of data governance include:
- Data Stewardship: Defining roles and assigning accountability for data management.
- Policy Definition: Establishing rules for data access, sharing, and usage.
- Data Quality Management: Continuously monitoring and improving data quality.
- Regulatory Compliance and Security: Ensuring adherence to industry regulations like GDPR, CCPA, or HIPAA.
How Synergy Between Data Engineering and Governance Drives Success
1. Enhancing Productivity Through Collaboration
When data engineering and governance work cohesively, they create an environment where data teams can thrive. Data engineers can focus on building high-performing pipelines without worrying about regulatory or policy compliance, as governance frameworks provide clear guidelines. This collaboration reduces time spent on manual processes, enabling teams to dedicate more resources to deriving actionable insights.
2. Boosting Efficiency with Automation
Automation is a game-changer when integrating data engineering with governance:
- Automated Quality Checks: Ensure that only accurate and reliable data enters analytics systems.
- Lineage Tracking: Automatically trace the origin and transformation history of datasets.
- Compliance Monitoring: Validate data processes against governance policies in real-time.
This streamlined approach eliminates redundant manual tasks, accelerates workflows, and reduces the likelihood of errors.
3. Ensuring Accuracy with Strong Frameworks
Data accuracy is non-negotiable for sound decision-making. A robust governance framework enforces consistent data definitions, validation rules, and maintenance protocols. By embedding governance principles directly into data pipelines, data engineers can ensure high accuracy. Regular audits and monitoring further reinforce reliability, offering stakeholders confidence in the data they rely on.
The Benefits of Integrating Data Engineering and Governance
- Improved Decision-Making: Reliable, high-quality data empowers teams to make informed choices quickly.
- Scalable Processes: Well-structured governance allows data engineering teams to scale operations without compromising quality.
- Enhanced Compliance: Adherence to regulatory standards mitigates risks and protects the organization from legal penalties.
- Cost Efficiency: Streamlined workflows reduce redundancies, saving time and resources.
Conclusion
The convergence of data engineering and data governance is no longer optional—it’s essential for organizations aiming to thrive in a data-driven world. By fostering collaboration between these disciplines, leveraging automation, and prioritizing data accuracy, businesses can empower their teams to operate at peak productivity and efficiency.
As the data landscape continues to evolve, embracing this synergy will be critical for innovation, growth, and long-term success.
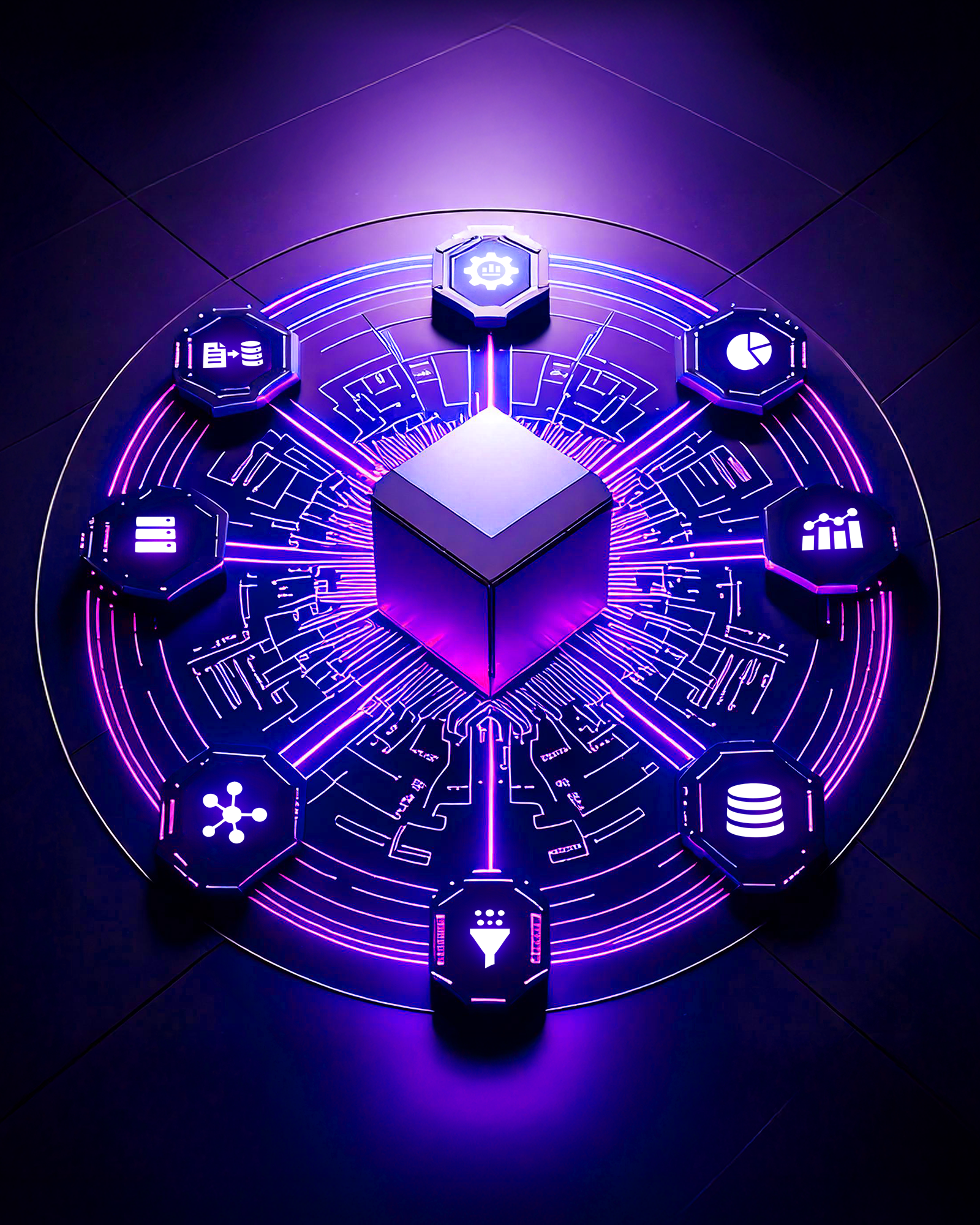
The Future of Data Monetization: Insights from Edgematics Group
In today's digital landscape, data transcends its traditional role as merely an asset; it has evolved into a significant revenue generator. The ability to convert raw data into actionable insights and profitable outcomes is reshaping the business landscape globally. However, the journey toward effective data monetization involves more than just data collection; it necessitates a comprehensive approach that includes data orchestration, engineering, governance, and machine learning.
The Future of Data Monetization: Insights from Edgematics Group
In today's digital landscape, data transcends its traditional role as merely an asset; it has evolved into a significant revenue generator. The ability to convert raw data into actionable insights and profitable outcomes is reshaping the business landscape globally. However, the journey toward effective data monetization involves more than just data collection; it necessitates a comprehensive approach that includes data orchestration, engineering, governance, and machine learning.
The Four Pillars of Data Monetization
1. Data Orchestration: Harmonizing Complex Ecosystems
Modern enterprises navigate a fragmented data environment, with information scattered across various sources, including cloud services, on-premises systems, and third-party platforms. Without effective orchestration, harnessing this data can be overwhelming.
Edgematics Group confronts this challenge with robust data orchestration solutions that integrate disparate data sources into a cohesive ecosystem, ensuring real-time accessibility and accuracy. Our solutions empower organizations to:
- Automate data workflows.
- Enhance operational efficiency.
- Enable seamless data integration across the enterprise.
2. Data Engineering: Building Strong Foundations
At the heart of any successful data strategy lies efficient data engineering. From data ingestion to transformation and storage, the ability to structure and prepare data effectively is crucial for advanced analytics and machine learning initiatives.
Edgematics focuses on creating scalable and robust data pipelines designed to:
- Process high volumes of data swiftly and accurately.
- Ensure scalability to accommodate growing business demands.
- Deliver clean, ready-to-use datasets for analytics and decision-making.
3. Data Governance: Ensuring Trust and Compliance
With increasing scrutiny on data privacy regulations, data governance has emerged as a critical priority. Beyond mere compliance, effective governance guarantees data quality and reliability, fostering trust among stakeholders.
Edgematics aids organizations in establishing strong governance frameworks that:
- Define clear ownership and accountability for data.
- Implement robust security protocols to safeguard sensitive information.
- Standardize data quality metrics to ensure consistent insights.
4. Machine Learning: Turning Insights into Action
Data monetization reaches its peak when organizations can predict trends, uncover hidden patterns, and automate decision-making processes. Machine Learning (ML) and Artificial Intelligence (AI) are instrumental in this transformation.
At Edgematics, we provide end-to-end AI and ML solutions tailored to meet business needs, enabling organizations to:
- Develop and deploy predictive models for customer behavior, market trends, and more.
- Automate repetitive tasks, allowing human resources to focus on strategic initiatives.
- Unlock real-time insights to drive proactive business decisions.
The Balancing Act: Overcoming Challenges
While each pillar plays a vital role, achieving synergy among them is essential for maximizing value. However, challenges such as siloed data, skill gaps, and scalability issues can impede progress.
Edgematics Group addresses these challenges through:
- Holistic Platforms: Our integrated solutions combine orchestration, engineering, governance, and ML into a single, streamlined platform.
- Expert Guidance: Our team of seasoned professionals collaborates with clients to design strategies tailored to their specific needs.
- Scalable Infrastructure: Utilizing cloud-native architectures, we ensure our solutions evolve alongside the business.
Looking Ahead: The Future of Data Monetization
The next frontier in data monetization will focus on aligning technological advancements with evolving business objectives. Success will be defined by focused learning models, real-time analytics, and improved interoperability.
At Edgematics Group, we are dedicated to driving innovation in:
- Personalized Data Solutions: platform that address industry-specific requirements.
- GenAI-Powered Insights: Utilizing advanced algorithms to uncover deeper opportunities.
- Sustainable Data Strategies: Ensuring long-term success through efficient and ethical data practices.
Empower Your Business with Edgematics Group
Edgematics Group is a niche, focused, all-in-data company that empowers enterprises with innovative solutions and trusted expertise. By combining consulting excellence with next-gen platforms, Edgematics helps organizations turn data into business value and achieve data-driven transformation at scale.
At Edgematics, we specialize in helping organizations turn data into business value by enabling:
✔️ Data Engineering & Governance – Building scalable, secure, and compliant data frameworks.
✔️ AI & Machine Learning – Driving AI-powered decision-making with real-time insights.
✔️ Agentic AI & Generative AI – Simplifying LLM adoption and unlocking enterprise-wide AI automation.
Data monetization is no longer a distant aspiration but an attainable reality with the right approach. Edgematics Group is here to guide your organization through every step of this journey. From streamlining operations to unlocking untapped potential, we provide the tools, expertise, and vision necessary to transform your data into a powerful business asset.
Are you ready to revolutionize your data strategy? Visit Edgematics Group today and take the first step toward unparalleled growth and innovation!
Edgematics – Turning Data into Business Value.
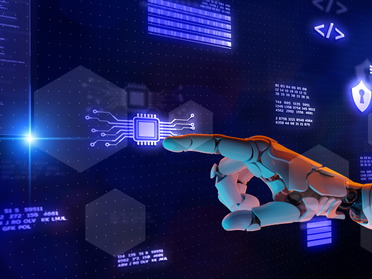
Connecting the Web: How PurpleCube AI Powers Seamless Data Integrations
Spider-Man, Marvel’s most iconic superhero, is celebrated for his incredible agility, adaptability, and—of course—his web-slinging abilities. Much like how Spider-Man’s web connects buildings, people, and possibilities, PurpleCube AI’s connectors empower enterprises to create seamless integrations across complex systems, weaving together data workflows that are efficient, reliable, and transformative.
Connecting the Web: How PurpleCube AI Powers Seamless Data Integrations
The Backbone of Automation: How Connectors Simplify Enterprise Workflows
Spider-Man, Marvel’s most iconic superhero, is celebrated for his incredible agility, adaptability, and—of course—his web-slinging abilities. Much like how Spider-Man’s web connects buildings, people, and possibilities, PurpleCube AI’s connectors empower enterprises to create seamless integrations across complex systems, weaving together data workflows that are efficient, reliable, and transformative.
The Role of Connectors: Building the Enterprise Web
In today’s data-driven world, businesses rely on an intricate network of systems, platforms, and applications. However, these systems often operate in silos, creating inefficiencies and bottlenecks. This is where connectors come into play, acting as the “webbing” that binds disparate systems together, enabling:
1. Seamless Data Integration: Just as Spider-Man effortlessly swings across the city, connectors allow enterprises to move data fluidly between platforms without disruption.
2. Real-Time Synchronization: Spider-sense helps Spider-Man react in real time; similarly, connectors enable instant data synchronization, ensuring that teams always have access to the latest insights.
3. Enhanced Collaboration: Much like Spider-Man teams up with other heroes, connectors bridge gaps between teams and tools, fostering cross-functional collaboration.
PurpleCube AI: The Ultimate Data Web-Slinger
PurpleCube AI’s GenAI-enabled platform takes the concept of connectors to the next level, offering data professionals:
Pre-Built Connectors for Popular Systems: From ERPs to CRMs and cloud warehouses, PurpleCube AI’s extensive library of connectors ensures quick and easy integration with enterprise systems.
Low-Code Customization: Tailor workflows without writing endless lines of code, just as Spider-Man adapts his web-slinging techniques for different challenges.
Scalable Architecture: Whether dealing with small datasets or large-scale pipelines, PurpleCube AI ensures your “web” can handle it all.
A Spider-Sense for Data
Spider-Man’s spider-sense warns him of danger and helps him navigate complex situations. Similarly, PurpleCube AI empowers data professionals with:
- Real-Time Alerts: Detect anomalies or bottlenecks in workflows, ensuring a smooth operation.
- Smart Recommendations: Leverage GenAI to optimize workflows, just like a superhero improving their strategy.
- Proactive Insights: Anticipate challenges and adjust data pipelines before they impact operations.
Weaving the Future of Data Workflows
Spider-Man’s story is one of connection, resilience, and responsibility. For enterprises, the lesson is clear: seamless integrations and powerful workflows aren’t just nice to have—they’re essential for staying competitive.
With PurpleCube AI’s cutting-edge connectors, you can become the hero of your data strategy, breaking down silos, streamlining processes, and delivering real-time insights. It’s time to spin a web of efficiency and innovation.
PurpleCube AI: Your Partner in Seamless Data Integration
Ready to harness the power of connectors? Join PurpleCube AI and build the ultimate web of enterprise efficiency.
Learn more about PurpleCube AI https://www.purplecube.ai/book-a-demo
.
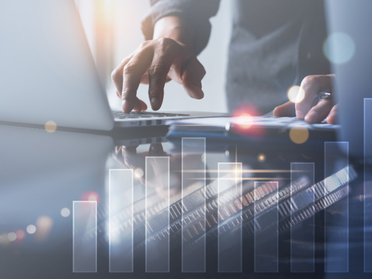
Unleash the Beast: Tapping into the Power of Unstructured Data
The untapped potential of unstructured data presents a unique opportunity for organizations to transform their operations and drive innovation. By adopting advanced technologies and strategies for harnessing unstructured data, businesses can unlock valuable insights that lead to informed decision-making and improved outcomes.
1. Abstract
In the age of information, unstructured data has emerged as a formidable force that businesses cannot afford to overlook. This eBook delves into the untamed potential of unstructured data, exploring its complexities, challenges, and the transformative power it holds for organizations.
From understanding the nature of unstructured data to leveraging advanced technologies like GenAI and Machine Learning, this comprehensive guide provides insights into how businesses can harness this data to drive innovation, enhance customer experiences, and optimize operations.
1.1. End Users
Data professionals such as data scientists, data engineers, data architects, data executives, and organizations from heathcare, telecommunication, banking and finance, retail, etc. are the end users who would benefit from this asset.
2. Introduction: The Untamed Potential of Unstructured Data
Unstructured data is often described as the wild frontier of the data landscape. Unlike structured data, which is neatly organized in rows and columns, unstructured data comes in various forms—text, images, audio, and video—making it challenging to analyze and utilize.
However, the sheer volume of unstructured data generated daily presents an unprecedented opportunity for businesses willing to tap into its potential. As organizations increasingly recognize the value of insights hidden within unstructured data, the need for effective strategies to harness this resource has never been more critical.
3. Why Businesses Can’t Ignore It Anymore
The digital transformation has led to an explosion of unstructured data, with estimates suggesting that it accounts for over 80% of all data generated today. Businesses that fail to recognize the importance of unstructured data risk falling behind their competitors. By leveraging unstructured data, organizations can gain deeper insights into customer behavior, market trends, and operational efficiencies. Ignoring this data is no longer an option; it is essential for driving innovation and maintaining relevance in a rapidly changing business landscape.
4. The Challenges of Harnessing Unstructured Data
While the potential of unstructured data is immense, harnessing it comes with its own set of challenges. Organizations must navigate the complexities of integrating unstructured data with traditional systems, ensuring data quality and governance, and addressing the volume, variety, and velocity of data—often referred to as the "Triple V Problem."
4.1. Volume, Variety, and Velocity: The Triple V Problem
The sheer volume of unstructured data generated daily can overwhelm traditional data processing systems. Additionally, the variety of data formats—from text and images to audio and video—requires diverse analytical approaches. Finally, the velocity at which unstructured data is generated necessitates real-time processing capabilities to derive timely insights.
4.2. Integration Complexities with Traditional Systems
Integrating unstructured data with existing structured data systems can be a daunting task. Traditional databases are not designed to handle the complexities of unstructured data, leading to potential data silos and inefficiencies. Organizations must adopt new technologies and frameworks to facilitate seamless integration.
4.3. Data Quality and Governance Issues
Ensuring the quality and governance of unstructured data is crucial for accurate analysis. Poor data quality can lead to misleading insights, while inadequate governance can expose organizations to compliance risks. Establishing robust data management practices is essential for leveraging unstructured data effectively.
5. From Chaos to Clarity: Techniques to Process Unstructured Data
To unlock the value of unstructured data, organizations must employ advanced techniques for processing and analysis. These include:
5.1. Natural Language Processing (NLP)
NLP enables machines to understand and interpret human language, allowing businesses to analyze text data from sources such as customer reviews, social media, and support tickets. By extracting sentiment, intent, and key themes, organizations can gain valuable insights into customer perceptions and preferences.
5.2. Computer Vision and Image Recognition
Computer vision technologies enable the analysis of visual data, such as images and videos. Businesses can leverage image recognition to identify patterns, detect anomalies, and enhance security measures. This technology is particularly useful in industries like retail and healthcare, where visual data plays acritical role.
5.3. Audio and Video Analytics
Audio and video analytics involve the examination of sound and visual content to extract meaningful insights. This can include analyzing customer interactions in call centers or monitoring video feeds for security purposes. By harnessing these technologies, organizations can improve customer service and enhance operational efficiency.
5.4. Text Analysis and Sentiment Mining
Text analysis involves extracting insights from unstructured text data, while sentiment mining focuses on understanding the emotional tone behind the text. Together, these techniques enable businesses to gauge customer sentiment, identify trends, and make data-driven decisions.
6. The Role of AI and ML in Unlocking Unstructured Data
Artificial Intelligence (AI) and machine learning (ML) are revolutionizing the way organizations analyze unstructured data. These technologies enable businesses to automate data processing, uncover hidden patterns, and derive actionable insights.
6.1. How AI Models Analyze Unstructured Data
AI models can be trained to analyze unstructured data by identifying patterns and relationships within the data. This allows organizations to gain insights that would be difficult or impossible to uncover using traditional analytical methods.
6.2. Machine Learning Pipelines for Continuous Learning
Machine learning pipelines facilitate continuous learning by allowing models to adapt and improve over time. As new unstructured data is ingested, AI models can refine their analyses, leading to more accurate insights and predictions.
6.3. Examples of AI-Driven Insights
Organizations that leverage AI to analyze unstructured data have reported significant improvements in decision-making and operational efficiency. For example, retailers can use AI-driven insights to personalize marketing campaigns, while healthcare providers can enhance patient care through predictive analytics.
7. Real-World Applications of Unstructured Data
The applications of unstructured data are vast and varied, with organizations across industries leveraging this resource to drive innovation and improve outcomes.
7.1. Enhancing Customer Experience with Sentiment Analysis
By analyzing customer feedback and sentiment, businesses can gain insights into customer preferences and pain points. This information can be used to enhance products, services, and overall customer experience.
7.2. Fraud Detection Using Behavioral Analytics
Unstructured data can be instrumental in detecting fraudulent activities. By analyzing patterns in customer behavior, organizations can identify anomalies and mitigate risks effectively.
7.3. Optimizing Operations with Document Digitization
Document digitization allows organizations to convert unstructured documents into structured data, enabling easier access and analysis. This can lead to improved operational efficiency and reduced costs.
7.4. Predictive Analytics from Social Media Feeds
Social media platforms generate vast amounts of unstructured data that can be analyzed for predictive insights. Organizations can monitor trends and customer sentiment to inform marketing strategies and product development.
8. Building a Scalable Unstructured Data Pipeline
To effectively harness unstructured data, organizations must build a scalable data pipeline that facilitates data ingestion, processing, and analysis.
8.1. Designing a Data Ingestion Framework
A robust data ingestion framework is essential for capturing unstructured data from various sources. This framework should support real-time data processing and ensure seamless integration with existing systems.
8.2. Tools and Technologies to Process and Store Data
Organizations must invest in the right tools and technologies to process and store unstructured data. This includes data lakes, cloud storage solutions, and advanced analytics platforms that can handle diverse data formats.
8.3. Automation for Real-Time Insights
Automation plays a crucial role in enabling real-time insights from unstructured data. By automating data processing and analysis, organizations can respond quickly to emerging trends and customer needs.
9. The PurpleCube AI Advantage: Simplifying Unstructured Data Orchestration
PurpleCube AI offers innovative solutions for organizations looking to simplify the orchestration of unstructured data. Our platform provides low-code tools for complex data integration, AI-driven data governance, and scalable solutions for enterprise automation.
9.1. Low-Code Tools for Complex Data Integration
Our low-code tools empower organizations to integrate unstructured data with minimal technical expertise. This accelerates the data integration process and enables teams to focus on deriving insights rather than managing data.
9.2. AI-Driven Data Governance and Compliance
PurpleCube AI’s solutions ensure that organizations maintain data quality and compliance. OurAI-driven governance framework helps organizations adhere to regulations while maximizing the value of their data.
9.3. Scalable Solutions for Enterprise Automation
Our scalable solutions enable organizations to automate data processing and analysis, driving efficiency and innovation across the enterprise.
10. Future Trends: The Growing Importance of Unstructured Data
As technology continues to evolve, the importance of unstructured data will only increase. Organizations must stay ahead of emerging trends to remain competitive in the data-driven landscape.
10.1. Emerging Technologies to Watch
Technologies such as advanced NLP, computer vision, and AI-driven analytics will continue to shape the future of unstructured data analysis. Organizations that embrace these technologies will be better positioned to leverage their data for strategic advantage.
10.2. AI’s Role in the Evolving Data Landscape
AI will play a pivotal role in the evolving data landscape, enabling organizations to automate processes, uncover insights, and drive innovation. The integration of AI into unstructured data analysis will become increasingly essential for success.
10.3. Predictions for Data-Oriented Organizations
Organizations that prioritize unstructured data will gain a competitive edge, driving innovation and enhancing customer experiences. The ability to harness unstructured data effectively will become a key differentiator in the marketplace.
11. Conclusion: Unleashing the Beast for Business Transformation
The untapped potential of unstructured data presents a unique opportunity for organizations to transform their operations and drive innovation. By adopting advanced technologies and strategies for harnessing unstructured data, businesses can unlock valuable insights that lead to informed decision-making and improved outcomes.
11.1. Key Takeaways for Data-Driven Success
- Unstructured data is a valuable resource that organizations must leverage to remain competitive.
- Advanced technologies such as AI and machine learning are essential for processing and analyzing unstructured data.
- Building a scalable data pipeline is crucial for effectively harnessing unstructured data.
11.2. Next Steps to Harness Unstructured Data
Organizations should assess their current data strategies and identify opportunities for integrating unstructured data into their workflows. Investing in the right tools and technologies will be key to unlocking the full potential of unstructured data.
11.3. Transform with PurpleCube AI
Are you ready to unleash the beast within your data? Contact us today for a free trial and discover how PurpleCube AI can help you dominate the data frontier. Together, we can transform your organization into a data-driven powerhouse.